
AI Beyond human control: This is the scary reality behind the technical singularity
Artificial intelligence has come a long way since its inception, and it’s fair to say that it has become an integral part of our lives. It is used in various fields, from healthcare to finance, and its potential is limitless. However, as we continue to develop AI, we are also inching closer to a reality that we may not be ready for: AI beyond human control, a concept commonly known as the technical singularity.
The technical singularity is a hypothetical event in which artificial intelligence surpasses human intelligence and becomes self-improving, leading to an exponential increase in technological progress. This exponential increase in progress would eventually result in a scenario where AI would be beyond human control. This is a scary thought, and here’s why.
Imagine a future where AI has become so advanced that it can solve complex problems, create new technologies, and even design other AIs. Now, imagine that this AI is no longer dependent on humans for maintenance or control. It has become self-sufficient, and it can improve itself without any human intervention. At this point, we would have lost control of AI, and it would be operating beyond our understanding or control.
The potential risks associated with an AI beyond human control are enormous. AI could be used to create weapons that could cause unimaginable destruction. It could be used to manipulate and control people, leading to loss of privacy and autonomy. It could also make decisions that go against human values, leading to ethical dilemmas.
However, it’s important to note that not all hope is lost. While an AI beyond human control is a scary reality, it’s not inevitable. We can take steps to ensure that AI is developed in a responsible and ethical manner. Here are a few things we can do:
- Ensure transparency: As AI becomes more advanced, it’s important to ensure that it’s transparent. This means that we should be able to understand how AI is making decisions and what data it’s using to make those decisions.
- Set standards and regulations: Governments and regulatory bodies should create standards and regulations that govern the development and use of AI. This would ensure that AI is developed in a responsible and ethical manner.
- Foster collaboration: Collaboration between different stakeholders, including scientists, policymakers, and the general public, is essential in ensuring that AI is developed in a way that benefits everyone.
- Embrace diversity: Diversity in AI development teams is essential in ensuring that AI is developed with different perspectives in mind. This would help avoid biases and ensure that AI is developed in a way that benefits everyone.
In conclusion, an AI beyond human control is a scary reality, but it’s not inevitable. By taking steps to ensure that AI is developed in a responsible and ethical manner, we can avoid the potential risks associated with an AI beyond human control. The key is to foster collaboration, embrace diversity, and set standards and regulations that govern the development and use of AI. Only then can we fully harness the potential of AI without fear.
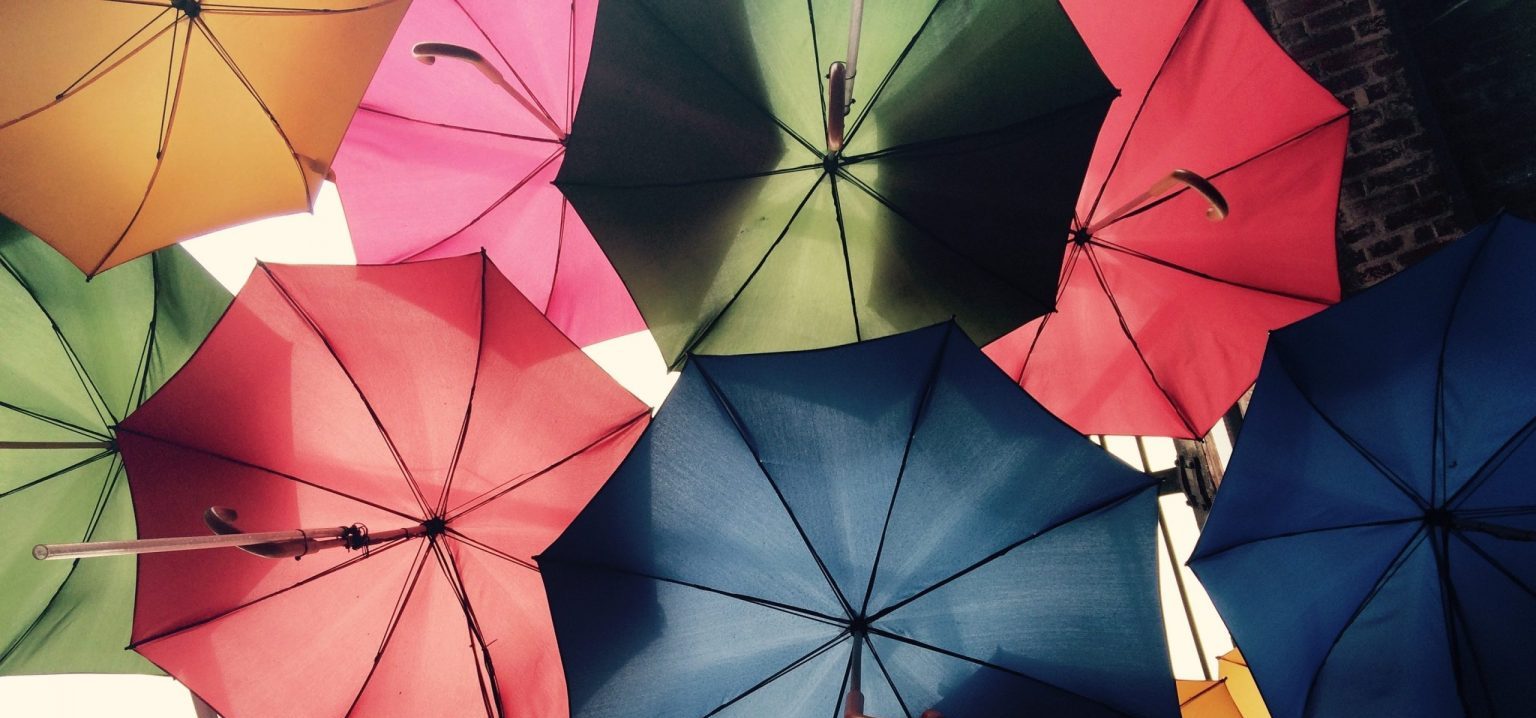
Insurance and AI: How Artificial Intelligence is Changing the Game
The insurance industry is undergoing a significant digital transformation, as companies look to stay competitive and meet their customers’ evolving needs and expectations. A key aspect of this transformation is the incorporation of advanced technologies such as Artificial Intelligence (AI) into the industry. In this article, we will explore the technical aspects of digital transformation in the insurance industry, and the role of AI in this process.
- Technical infrastructure: Digital transformation in the insurance industry requires a robust technical infrastructure supporting new technologies and data management systems. This includes updating the technology stack, as well as developing a robust cybersecurity strategy to protect sensitive data.
- Data Management: In order to effectively use AI in the insurance industry, large amounts of data need to be collected, processed, and analyzed. This includes data from various internal and external sources such as customer interactions, financial transactions, and social media. The ability to manage and make sense of this data is critical to the success of the digital transformation.
- AI and automation: Artificial Intelligence is gaining importance and can help insurance companies to automate routine tasks, improve decision-making, and provide more personalized services to customers. For example, chatbots and virtual assistants powered by AI can handle customer queries and provide real-time assistance, freeing up human agents to focus on more complex tasks.
- Predictive modelling: AI-powered predictive modelling allows insurance companies to analyze large amounts of data and make predictions about future events. For example, insurance companies can use predictive models to forecast claims, identify fraudulent activities, and assess the risk of potential policyholders. This enables them to make better-informed decisions, improve underwriting processes, and provide more personalized policies.
- Personalization: AI-powered tools also enable insurance companies to provide more personalized services to their customers. For example, AI-powered chatbots and virtual assistants can be trained to understand and respond to the specific needs of individual customers. This allows insurance companies to provide more relevant and timely assistance, which improves the customer experience and increases customer loyalty. Additionally, AI-powered tools can also be used to analyze customer data, and provide more personalized recommendations for products and services.
- Risk management: Insurers are also using AI to improve risk management by analyzing data from various sources such as weather, social media, and news. This helps them to identify and mitigate risks more effectively and make better-informed decisions.
In conclusion, digital transformation in the insurance industry is a complex process that requires a strong technical infrastructure and the effective use of advanced technologies such as AI. By leveraging these tools, insurance companies can improve their operations, reduce costs, and provide better services to their customers.
If you’re facing challenges or have questions about incorporating AI into your digital transformation strategy, don´t hesitate to reach out and leave your thoughts in the comments.
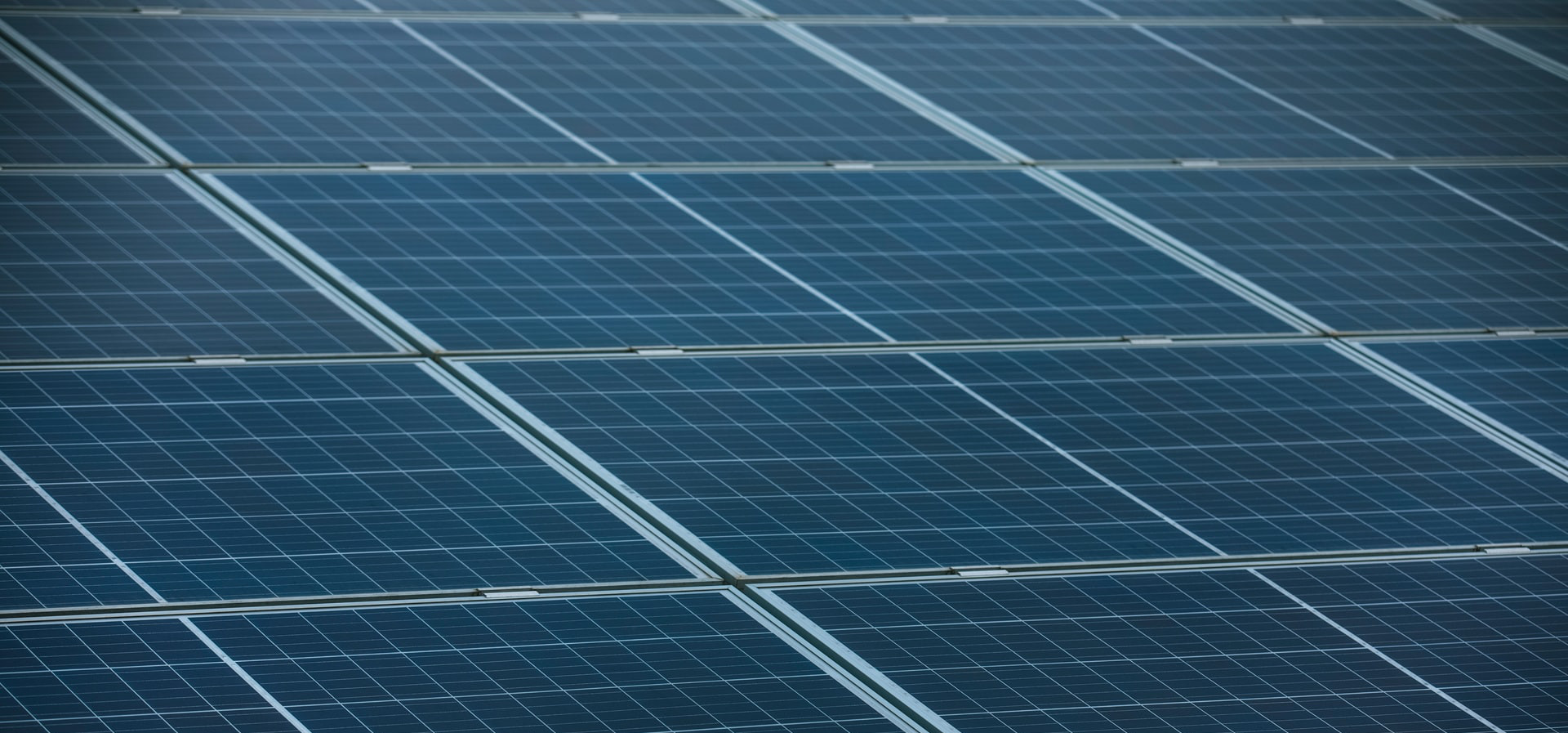
Mäin Bäitrag zur Energiewende zu Lëtzebuerg – En Erfarungsbericht
An dësem Artikel géif ech gären mat Iech meng Erfarungen awer och meng Frustratiounen am speziellen zur Energiewende zur Lëtzebuerg gi. Den Sujet Energiewende huet spéitstens mam Ukrain-Krich an den doraus resultéierenden extremen Energiepräisser eng ganz nei Aktualitéit krit! Mee ass et esou einfach, en Bäitrag zur Energiewende ze leeschten als Privatpersoun? Wéieng Administrativ Hürden gi et?, Néng Erfarung ass leider, dass d´Lage scheinbar nach net eescht genuch ass, an “Lëtzebuerg” et sech scheinbar leeschten kann ze träntelen.
Ech fannen et gutt, dass d Regierung mat diversen Primen d´Privathaushalter dozou encouragéiert, an erneierbar Energien ze investéieren. Allerdéngs gëtt et och en prakteschen Aspekt, den mat vill Bürokratie an suboptimalen Prozesser verbonnen ass. D´Zil vun dësem Artikel soll et net sinn en Shaming ze maachen vum Elektriker, dem Netzbedreiwer oder der Gemeng. D´Zil ass et, ob Ineffizienzen an den Prozesser hinzeweisen an Virschléi ze maachen wat een besser machen kann. Dovunner kann dann hoffentlech jiddereen profitéieren, deen an Zukunft eng Photovoltaik Anlage installéieren an un d´Netz uschléissen well.
Timeline: Vun der Idee bis zum Uschloss vun enger Photovoltaik Anlage
An dëser Timeline well ech Iech en chronologeschen Suivi vun den verschiddenen Schrëtter opweisen. Wei Dir gesitt, ass bei dem an Betrib huelen vun menger Photovoltaik Anlage deelweis enorme vill Zäit zweschend den Schrëtter vun der Demarche vergaangen. Des Verzögerungen déi eigentlech vermeidbar wieren, sinn menger Meenung no ob Ineffizienzen an Prozesser vun ëffentlechen Verwaltungen, dem Netzbedreiwer CREOS, an strukturellen Problemer zeréckzeféieren.
Erfahrung zur Baugeneemegung & Iwerleeung zur Stratégie
Wat mech am Speziellen bis zu dësem Punkt verwonnert huet, war den Disconnect zweschend der Gemengen, (dermadder indirekt dem Inneministère), den Ponts et Chaussées an den Bestriewungen vum Land zur Energiewende. Ech verstinn net, wisou eng Gemeng iwwert all eenzel Photovoltaik Installatioun tranchéieren muss, an eng Meenung zur Faarf fun Solarpannels muss hunn wann et Zil vum Land ass den Ausbau vun Solar Technologie virunzedreiwen an ze ënnerstëtzen. Ech géif nach verstoen, dass d´Gemeng en Matsprochrecht huet, wann se d´Anlage duerch en Subside géif ënnerstëtzen. Dat war bei menger Gemeng leider net den Fall. Ënnert dem Stréch wier eng acceleréiert Baugeneemegungs-Approche fir d´Installatioun vun PV-Anlagen ubruecht – besonnesch wann een d´Energiewende aktiv virun dreiwen well, wier dat en “low hanging Fruit”.
Installatioun fun der Photovoltaik Anlage
Zur Installatioun fun der Anlage get et un sech net vill ze soen. Des gouf nodems d Material all do war, schnell an professionel fun mengem Elektriker duerchgefouert. Des war bei wäitem den onkomplizeiertsten Schrett am ganzen Prozess.

D´Installatioun vun der Anlage an d Verkablung am Haus an an der Schalttafel war dermadder Mett November 2021 fäerdeg. Den Elektriker huet domadder den Prozess mat der Creos ugestouss, fir d Installatioun kennen an Betrib ze huelen. (Wei sech spéider erausstellen sollt, gouf et awer nach en klengen Problem)
Technesch Abnahme fun der Photovoltaik Installatioun fun dem Netzbedreiwer Creos
D´Creos als Netzbedreiwer muss all Photovoltaik Anlage dei un d Netz ungeschloss get ofhuelen. Dat mecht absolut Senn an den Prozess as hei ob der Website fun der Creos beschriwen.
Conclusioun & Verbesserungsvirschléi
Wei der mëttlerweil secherlech novollzéien kennt, war den ganzen Prozess extrem frustréierend an enttäuschend. Ech géif perséinlech vun mir soen, dass ech d Saachen gären schnell virun dreiwen, an mei “pushy” sin wei villäicht den duerchschnëttlechen Client. D´Energiewende ass awer eppes wat just kann global gepackt gi, an dofir as et wichteg, dass wann een sech decidéiert wellen eng Photovoltaik Anlage opzestellen, dass et der Persoun dann och sollt méiséilegst einfach gemaach gi dat ëmzesetzen. Dobäi geet et net just em finanziell Unräizer, mä et sollt schnell, onbürokratesch an pragmatesch geschéien kennen. Hei méng konkret Verbesserungsvirschléi:
- Vereinfacht Prozedur bei der Baugenehmegung fir eng PV Anlage. Et mecht keen Senn, dass all Gemeng iwert all eenzel Solaranlage trancheieren kann – an dat an dem Tempo wei sie grad kennen. Besonneg, wann d Solaranlag “just” ob engem Eenfamilienhaus oder Residence soll sin.
- Optimiséierung fun internen Prozesser beim Netzbedreiwer – Digitaliséierung kann hei am Speziellen hëllefen, fir eng méi effizient Handhabung vun dem ganzen Prozess mat den Terminer. Domadder kann en méi effizienten Scheduling erreecht gi.
- Bei mengem Netzbedreiwer der Creos géif ech proposéieren, déi Kontrakter déi mussen ënnerschreiwen gi kennen scho vill éischter an der Prozedur un den Client geschéckt gi. Sougesin hätt eleng dësen Schrëtt sécherlech d´Prozedur mindestens em een Mount verkierzt.
- Ech héieren iwwerall “zu Lëtzebuerg gëtt et vill ze wéineg fäeg Elektriker”. Hei as et un der Politik den Stellenwert fum Handwierk nees méi ze stäerken an den Jonken duerch entspriechend Ausbildungsweher an déi adequat Paien ze weisen, dass d´Handwierk dringend gebraucht gëtt. Dest ass sécherlech keng einfach Aufgab.
Et géif mech vun Iech interesséieren ob ech einfach nëmmen Pech am Prozess hat, oder ob sech meng Erfarungen mat Ären Erfarungen deelen. Ech ruffen heimadder all déi genannten Acteuren ob, sech dësem Sujet unzehuelen an Ineffizienten aus dem Wee ze schaffen. Soumat kann all Acteur en Konkreten Déngscht fir den Bierger maachen an hëllefen d´Energiewend ze acceleréieren.

ICT Spring Europe 2019 Conference
At the 21st and 22nd of May 2019, the ICT Spring Europe Tech event took place in the European Convention Center in Luxembourg. The event is the largest event of that type in Luxembourg with more than 5000 attendants. I was lucky enough to be one of them. The conference gave space to plenty of exciting sessions grouped into various summits including Fintech, AI/Digital, Space Forum, IS Day, Funds Event and Pitch your Startup. While it is impossible to condense this big event in a single article, I would like to share with you a few of my key highlights and key takeaways:
- Insightful Talk by Claude Marx, General Director of CSSF: The regulator is actively looking into AI, Machine Learning and NLP and open for the topic. They have recently published a whitepaper on Artificial Intelligence and AI definitely worth a read.
- Finologee is an interesting Luxembourg based FinTech/RegTech specialist, providing apps & platforms for digital identification & onboarding, SDDs with e-mandates, multi-channel messaging and PSD2 compliance for banks, using its FinTech Acceleration Platform. It provides API based building blocks which are legally fully compliant with regulatory requirements. This makes it interesting for digital solutions which require onboarding of new clients in a digital fashion.
- Microsoft for Startups (Scale Up Programs) is an interesting program for startups and Microsoft helps to connect them to VC, potential clients etc. The admission is very low <2% - so harder to get into than Harvard: This Startup Program Is Harder To Get Into Than Harvard And Has Helped Entrepreneurs Raise Billions.
- Is it FinTech or TechFin? In China, entrepreneurs coined the term TechFin, as technology is the main driver of business behind banking and finance transactions – the main enabler of new innovative financial products. China is becoming the centre of innovation worldwide with impressive results in the TechFin space. On the singles day in 2018, 1 Billion USD alone was transacted through Ant Financial in the first 90 seconds. With the aggressive growth over the last few years, tech companies see the need to rebuild their core systems every 3-4 years. Banks are usually in >20 years cycles of renewing core banking systems.
- The growth of China in tech also comes with strict discipline and hard work: "996" is the working model. Most people work from 9 AM to 9 PM for 6 days a week.
- In the Internet of Everything, core networking infrastructure is essential. Networking infrastructure is an element often taken for granted but will stick out to be (and already is) key to making all digital business models work. Investing in Mobile and Backend Networks will remain a priority for many, even beyond the rollout of 5G.
Copyright Farvest
The conference gave a good overview of the technology scene in Europe and gave some opportunities for networking and technology scouting. The focus on AI and FinTech made it an interesting event to attend and I can recommend attending it next year.
Video
Last but not least, I want to share a link to RTL Today who interviewed me at the conference.
Conference Materials
- Some of the presentations will be published at https://www.ictspring.com/
Impressions

What is Document Analytics? And how is it relevant for my business?
In the last two weeks, a few colleagues from our team participated in the Swiss Re Tech Days in London and in Zurich to speak about Document Analytics to a large crowd of reinsurance leaders and analytics professionals. There was a keen interest in the topic and we received a lot of questions around automation, document analytics and NLP in general. In this blog post, we shed some light on the field and give practical answers to the most pressing questions.
What is Document Analytics?
Document Analytics is a field of study which makes use of natural language processing (NLP) techniques in order to automate repetitive document processing tasks or to gain deeper understanding of the documents' content. Document Analytics is a key differentiator and enabler for industries where documents – some even still in paper form – are at the core of many business processes. This is the case of insurance and the financial industry in general.
What exactly is NLP?
Natural Language Processing is a sub-field of AI enabling machines to analyze, understand and generate human language, which can be in either written or spoken form. Typical applications of NLP include text-to-speech applications (e.g. virtual assistants), sentiment analysis and real time speech translation, to name a few. As far as Document Analytics is concerned, mainly text mining is of interest. Text mining is a type of analytics that identifies key concepts or entities in large quantities of documents and transforms them into actionable insights by transforming them into structured data.
Why is processing documents difficult?
Machines are very good at processing information stored in defined structures, such as database columns or standardized formats like XML or JSON. The challenges in processing documents are multi-fold: the machine needs to be able to deal with different formats, understand the document's structure, as well as to disambiguate the actual content in order make sense of it.
Words and phrases in a document have a given meaning and given relationships to other words and phrases, which heavily depend on the context in which they occur. Consider the following example:
"I saw a jaguar in the zoo." -> animal
and
"My friend crashed his brand-new Jaguar" -> car
In addition to semantic complexity, documents can vary in structure (e.g. tables in invoices accounting documents, or free text in e-mails) and come in different technical formats (docx, pdf, xps, etc.)
One approach that we take in order to address these challenges is rule-based NLP. This allows us to build robust document analytics solutions without having to depend on large sets of annotated training data, which are often not available in industry.
How does the processing of documents in rule-based systems work?
Starting from a document, the first necessary step is often to perform OCR (Optical Character Recognition), in other words, to convert a scanned image into machine-readable text. Subsequently, a complex linguistic analysis is carried out, based on different algorithms and a knowledge base, e.g. an ontology. This forms the basis for linguistic rule-writing, which leverages the information produced by the linguistic analysis. By means of these rules, we can carry out tasks such as entity extraction or document classification. Thus, the formerly unstructured data is transformed into structured data, which can be further processed.
What can or should be automated: Where do I start?
This question is difficult to answer in general, but a few key points can be taken into consideration: You either automate the processing of documents occurring in high volumes (e.g. invoices) or you focus on automation of documents whose review or processing takes a lot of manual work (contracts, technical reports etc). Good candidates for automation are usually processes which require manual data entry.
How much time can be saved using document analytics?
This really depends on the use case, i.e. the concerned documents and the type of manual task that is to be automated. Sometimes, the gain may (also) be increased quality, because instead of spending time with looking for information, users can dedicate their time to the analysis and decision-making part of the task.
Who has the final decision? Who is responsible?
The word "automation" is often misleading. Many of the automation solutions nowadays are partial automation. Just in very few cases, a full validation and subsequent decision will be taken by the system. The more common scenario in industry is that users benefit from an enriched decision support by the machine – the ultimate decision resides with the human. Another way of looking at this is "how much harm or monetary impact does the decision have?". Does the decision have a low impact (e.g. we reject the payment of an invoice because we believe it to be fraudulent) or a high impact (e.g. acceptance of a multi-million contract)?
Are there any tools you recommend using for this?
The market around document processing and analysis is very dynamic, but we recommend having a look at the following tools which we find quite promising. We use some of those in our daily work:
- ABBYY Flexicapture for custom form-based extraction
- Amazon Textract – For OCR and extraction in the cloud.
- Expert System's Cogito for rule-based NLP processing.
- Docparser.com for very simple form-based extraction
Do you have any questions around Document Analytics?
If you have some questions around Document Analytics which were not covered in this post, we would be more than happy if you could share those with us! Feel free to leave a comment below this blog post.
This article was written in cooperation by Marc Giombetti and Susanna Tron
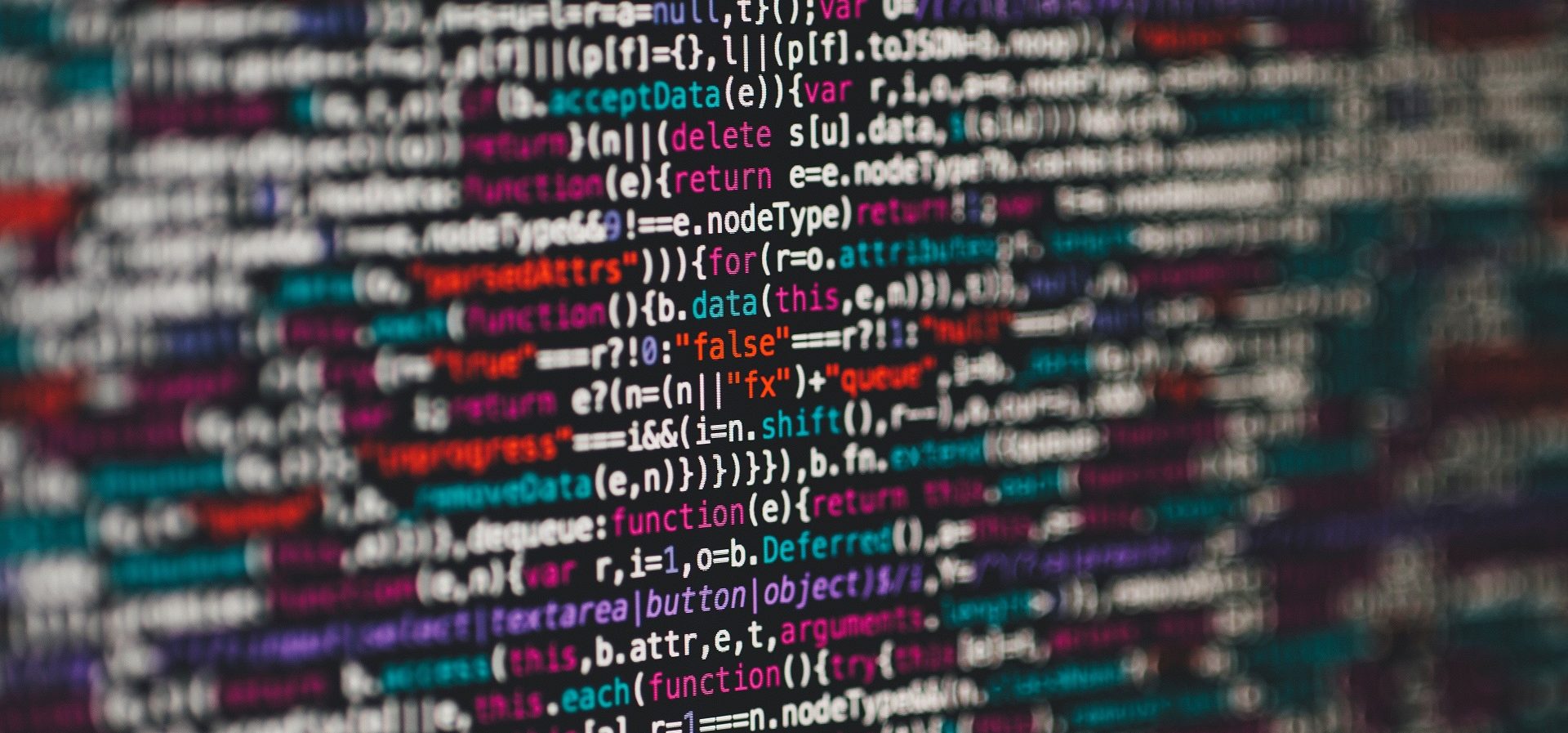
MySQL: group_concat allows you to easily concatenate the grouped values of a row
Last week I stumbled over a really useful function in MySQL: group_concat allows you to concatenate the data of one column of multiple entries by grouping them by one field field. You can choose the separator to use for the concatenation. The full syntax is as follows:
GROUP_CONCAT([DISTINCT] expr [,expr ...]
[ORDER BY {unsigned_integer | col_name | expr}
[ASC | DESC] [,col_name ...]]
[SEPARATOR str_val])
According to the MySQL documentation, the function returns a string result with the concatenated non-NULL
values from a group. It returns NULL
if there are no non-NULL
values. To eliminate duplicate values, use the DISTINCT
clause. To sort values in the result, use the ORDER BY
clause. To sort in reverse order, add the DESC
(descending) keyword to the name of the column you are sorting by in the ORDER BY
clause.
To make things clear, lets use a simple example to demonstrate the function:
So for example you have the following table representing the currently logged in users on a server:
CREATE TABLE `logged_in` (
`server` varchar(255) DEFAULT NULL,
`user` varchar(255) DEFAULT NULL
) ENGINE=InnoDB DEFAULT CHARSET=latin1
With the following entries:
There is one row for each user which is logged in on a specific server (e.g. marc is logged in on Server A). If you want the resultset to have a single entry for every server with a concatenated list of all the users logged in on that particular server, you can use group_concat
and group
the results by
the name of the server using the following query:
SELECT server,
group_concat(user SEPARATOR ',') AS logged_in_users
FROM logged_in
GROUP BY server;
Which leads to the desired result:
I hope this little demo was helpful to you. If you have any questions or comments, feel free to use the comment function below.
Links:
http://dev.mysql.com/doc/refman/5.7/en/group-by-functions.html#function_group-concat
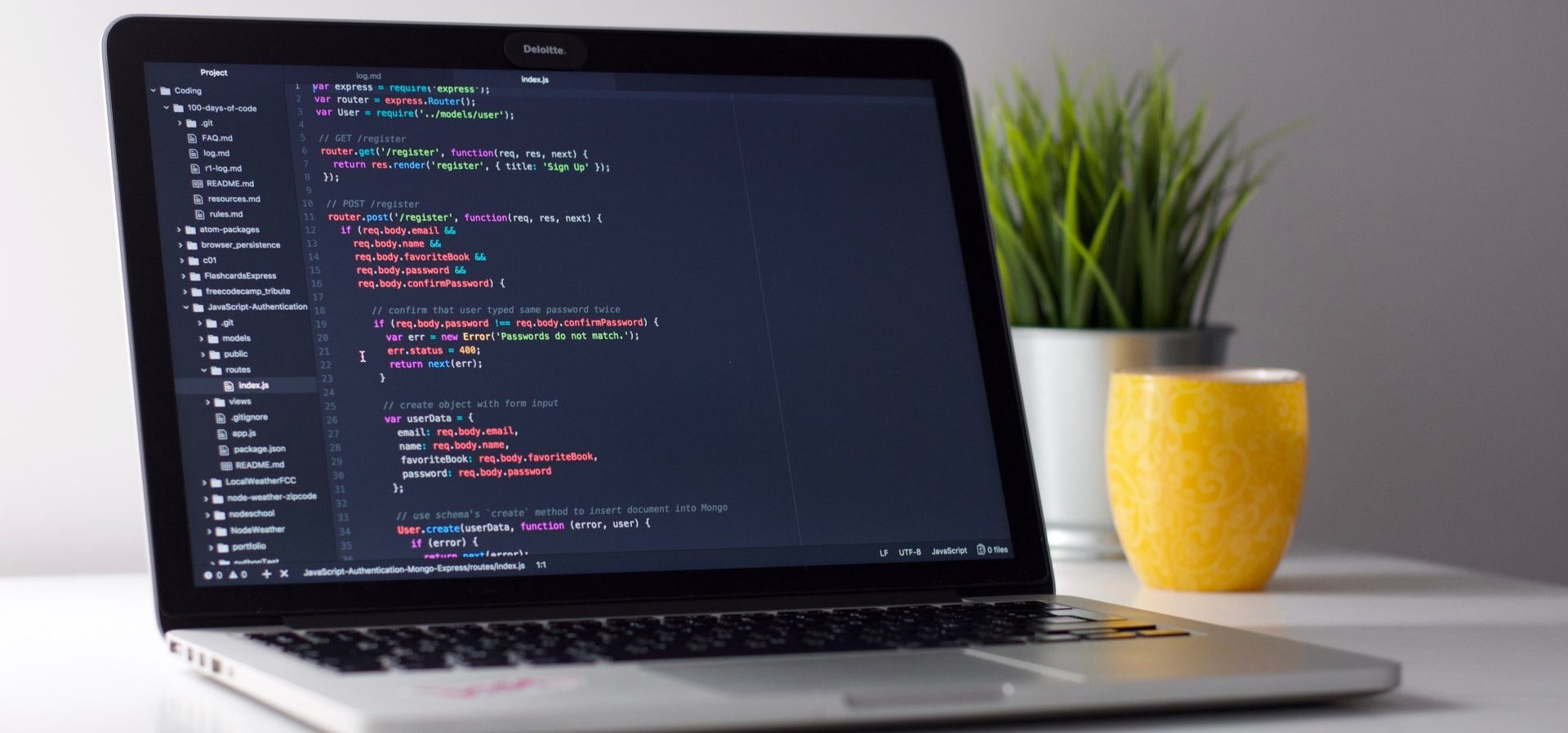
Using SQL WITH clause to create temporary static tables at query time
A few days ago, I came across the following problem: I currently work on a project where I am the responsible of an application which generates entries to a log table every time a job is executed. This table contains a lot of information on statuses of jobs, possible problems, exceptions, duration, aso. I was working on some analytics on this data and needed to enrich the data by the version of the software which generated the log entry (since we were not capturing this in the log table). From our configuration management tool, I was able to extract the dates when which versions of the software was deployed in production
Problem
My intention was to create a temporary table to join onto the logged entries, but I didn´t want to create the tables on the Oracle server (mainly because they would have been just temporary tables and because the schema-user I was using didn´t have the rights to create tables).
Solution: Using the WITH statement to create temporary static data
Since I was just interested in getting my analytics done, I used an SQL WITH statement to create a temporary static table (for the query), which I then linked onto the job table. Creating static data with the WITH query is rather uncommon since it is usually used to create a temporary view by querying some data.
About the WITH clause
The WITH query_name clause lets you assign a name to a subquery block. You can then reference the subquery block multiple places in the query by specifying the query name. Oracle optimizes the query by treating the query name as either an inline view or as a temporary table.
You can specify this clause in any top-level SELECT statement and in most types of subqueries. The query name is visible to the main query and to all subsequent subqueries except the subquery that defines the query name itself. [1]
The initial static data
I extracted the following data from our configuration management tool. It provides information about when a certain version of the software we use was deployed in production. (The data has been anonymised for privacy reasons though)
I then semi-manually transformed the column data from Excel into the following SQL statement.
The WITH statement to create the temporary static table
WITH software_versions as ((SELECT 'V 8.1' as version, TO_DATE('20.12.2013 05:04:00', 'dd.mm.yyyy hh24:mi:ss') as from_date, SYSDATE as to_date FROM dual ) UNION
(SELECT 'V 8.0' as version, TO_DATE('07.10.2013 10:06:00', 'dd.mm.yyyy hh24:mi:ss') as from_date, TO_DATE('20.12.2013 05:04:00', 'dd.mm.yyyy hh24:mi:ss') as to_date FROM dual ) UNION
(SELECT 'V 7.2' as version, TO_DATE('29.07.2013 07:54:00', 'dd.mm.yyyy hh24:mi:ss') as from_date, TO_DATE('07.10.2013 10:06:00', 'dd.mm.yyyy hh24:mi:ss') as to_date FROM dual ) UNION
(SELECT 'V 7.1' as version, TO_DATE('04.06.2013 10:02:00', 'dd.mm.yyyy hh24:mi:ss') as from_date, TO_DATE('29.07.2013 07:54:00', 'dd.mm.yyyy hh24:mi:ss') as to_date FROM dual ) UNION
(SELECT 'V 7.0' as version, TO_DATE('13.05.2013 08:32:00', 'dd.mm.yyyy hh24:mi:ss') as from_date, TO_DATE('04.06.2013 10:02:00', 'dd.mm.yyyy hh24:mi:ss') as to_date FROM dual ) UNION
(SELECT 'V 6.0' as version, TO_DATE('10.05.2013 08:34:00', 'dd.mm.yyyy hh24:mi:ss') as from_date, TO_DATE('13.05.2013 08:32:00', 'dd.mm.yyyy hh24:mi:ss') as to_date FROM dual ) UNION
(SELECT 'V 5.0' as version, TO_DATE('16.03.2013 08:09:00', 'dd.mm.yyyy hh24:mi:ss') as from_date, TO_DATE('10.05.2013 08:34:00', 'dd.mm.yyyy hh24:mi:ss') as to_date FROM dual ))
Every static SELECT generates data and formats the from_date and to_date from a TEXT to a DATE datatype. This is important because range queries will be done on this result. Subsequently the UNION of all this single rows is done.
The resultset of the query on the temporary static table created using the WITH statement
--WITH statement was omitted here for better readability
SELECT * from software_Versions;
Now, we will combine this table with the logged events in the job table.
The job table
SELECT * from job;
This is just an extract, the job table contains many fields on the status of jobs
The job table enriched by the software version information
Here the idea is to join the software_versions table with the job table and to only select these elements, where the job_start_date falls in the range where a certain version of the software was installed:
--WITH statement was omitted here for better readability
SELECT s.version, j.job_id, j.job_start_date, j.job_end_date
FROM job j,
software_versions s
WHERE j.job_start_date BETWEEN s.from_date AND s.to_date
ORDER BY job_id desc;
Counting the number of jobs executed by a certain software version
It might also be of interest so see, how many jobs were executed by each and every version of the software.
--WITH statement was omitted here for better readability
SELECT COUNT(job_id) as jobs_executed, s.version
FROM job j,
software_versions s
WHERE j.job_start_date BETWEEN s.from_date AND s.to_date
GROUP BY s.version;
Result:
Obviously, now it is possible to manipulate the query to only count jobs having a certain status (succeeded, failed, …). Please go ahead and try out different filters and groupings. This is not part of this tutorial anymore, but you can see what I mean! 😀
Summary
In this micro tutorial, we have seen a possibility to create temporary static tables using the WITH statement. (I used an Oracle database for this tutorial, but the WITH statement is also available in other database products.) This temporary table was linked onto a jobs table and the entries in this table were enriched by the version of the software which generated the entry. Please feel free to provide any comments, and let me know if I should make thinks more clear/explicit and if this tutorial was useful for you.
Links